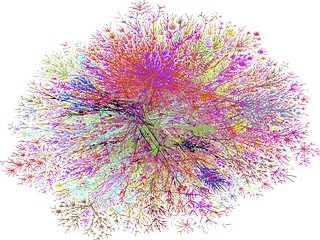
Structural inequality has been part of the Internet since its inception. According to United Nations Economic and Social Commission for Western Asia (UNESCWA), structural inequality specifically refers to inequality that is systematically rooted in the normal operation of social institutions which is unfair or bias differences that exists between different population groups in a given society. This unfair distinction is rooted in social practice, laws and regulations, government policies or politics, and ultimately has an impact on social politics and economics which has aggravated the gap between the rich and the poor and political turmoil. The early manifestations of this structural inequality are usually well-trained and well-educated men in the workplace, the majority are white and middle-class or upper-middle class (Lusoli & Turner, 2021). While structural inequality can be seen and is manifested in the society quite frequently, the development of the Internet has further exacerbated this inequality.
Racial discrimination – Spreading on the Internet.
1. Prejudices that continue to this day.
The treatment of Africans as slaves and their sale in the West for around 400 years has led to deaths of thousands of Africans (Martin, 2010). Even though slavery was abolished in America by President Lincoln in 1865, the remnants of racial discrimination can still be found within the American society and hence has made a deep impact on the minds of the people (Martin, 2010).
For instance, the recent incident that led to the death of an African American man George Floyd, depicted the Black person being crushed to death by a white policeman for no reason (Barrie, 2020). This incident has raised spark in both the real and virtual word, with the upsurge of the “Black Lives Matter” movement. In offline life, metropolises such as Los Angeles, New York, Sydney, Melbourne etc. have turned into centres for the “Black Lives Matter” parade. Besides, influential celebrities such as Taylor Swift have raised their voice against such racial discrimination on their social media platforms (Barrie, 2020). George Floyd’s death brought the focus of structural inequalities that exist in the so called ‘post-modern’ world.
2. Intangible influence.
The structural inequalities that exist in the society, can also be seen on the internet. Noble (2018) believes that the structural biases and stereotypes in search engines, can be seen in the advertisements showcasing Black girls on search engines, who can be seen in a lot of sex-related information. Another example is the racial discrimination in Uber’s facial recognition, which caused some Blacks to be fired for misrecognition, the App Drivers and CouriersUnion (ADCU) in the United Kingdom filed a lawsuit against Uber, regarding the same, in 2021. The union believed that Uber’s facial recognition system was less accurate while identifying people of colour (ADCU, 2021). Furthermore, the ” Black Lives Matter ” movement has brought the racial controversy in facial recognition to a climax. Both Microsoft and Amazon in 2020, announced that they will cease providing facial recognition technology to the police, and IBM directly stated that it would terminate its facial recognition business. Additionally, only a few members of the European Parliament are people of colour, while all EU commissioners are white (Stevens & Keyes, 2021). The existence of discriminatory algorithms and policies also indicates the lack of diversity among policymakers as well.
The Internet—A “Projector” of Sexism?

1. Low usage rate and high victimisation rate.
to the latest ITU estimate (2017), women around the world are 12% less likely to use the Internet than men. Mobile phones are considered an indispensable part of contemporary life, the usage of mobile phone differ between genders (Mariscal, Mayne and Aneja, 2019), this is one of the important reasons for sexism on the Internet. In addition, the South Korean Nth Room case refers to the establishment of multiple secret chat rooms on the social software Telegram (Telegram has been a breeding ground for crime since its establishment in 2013 because its slogan is “Taking back our right to privacy”) to illegally share photos and videos of women in order to threaten them (including underage girls) and use them as sex slaves. Research shows that 1 out of every 100 men in South Korea has seen the video of the Nth room (Joohee & Chang, 2021).
2. There is more “him” in the algorithm.

Sexism is quite evident on the web. Search engines have prejudices females of colour and different ethnicities as it exposes the commercialisation and sexualization of female identities and images within search engines (Noble, 2018). Even though, Google advertises that there is no gender discrimination, gender discrimination is deeply ingrained within Google. For instance, if the users search for suits in Google Images, most of the pictures of executives are related to men. Furthermore, netizens have long discovered that when a user searches for the keyword “her”, Google will prompt the user towards a typing mistake and whether they want to replace it with “him” (Prates, Avelar, and Lamb, 2020). Likewise, such problems also exist in Google Translate, and the translation is often first translated into “him” instead of “her”. Implying that Google Translate depicts a strong male default tendency, especially for fields that are usually related to gender imbalance or stereotypes.
“Potential exists for artificial intelligence to detect and embed discriminatory bias in human behaviour.”–Catherine Hanrahan
Hanrahan (2020) claims that large-scale recruitment websites like seek usually use AI algorithms to help employers choose more suitable candidates, but these algorithms tend to favour men over women in the job market. Even if female applicants match more closely with the keywords, the algorithm still prioritises male resumes. The above reasons indicate that these algorithms have reinforced unconscious gender bias within recruitment (Hanrahan, 2020). However, the fundamental reason behind this is also obvious — the stereotype of gender differences invisibly affects the programmers who write algorithms. Many artificial intelligence standards do not fully integrate gender perspectives. One such example is the Montreal Declaration on the Responsible Development of Artificial Intelligence lacks to mention gender perspectives, and AI4People’s good artificial intelligence social ethics framework only mentions gender only once (Université de Montréal 2018; FloridI et al., 2019).
Do structural inequalities arise from the Internet?
The answer is obviously no. Both racial discrimination and gender discrimination existed before the Internet, and stereotypes were formed in the minds of the public (Prates, Avelar, and Lamb, 2020). However, with the rapid popularity of Big Data, major companies are aiming to collect more user data. For example, Google, Apple, Amazon, and other platform services and their overall design is centred around data flow (Van Dijck et al., 2018). As a result, it is more and more convenient to publish and receive information, and the Internet has gradually become a catalyst. Internet users from all over the world could intensify the discussion about these structural inequality phenomena. The Internet can thus, be called a double-edged sword. Nonetheless, it also suggests that these discrimination issues on the Internet can be improved as well.
How to reduce inequality?
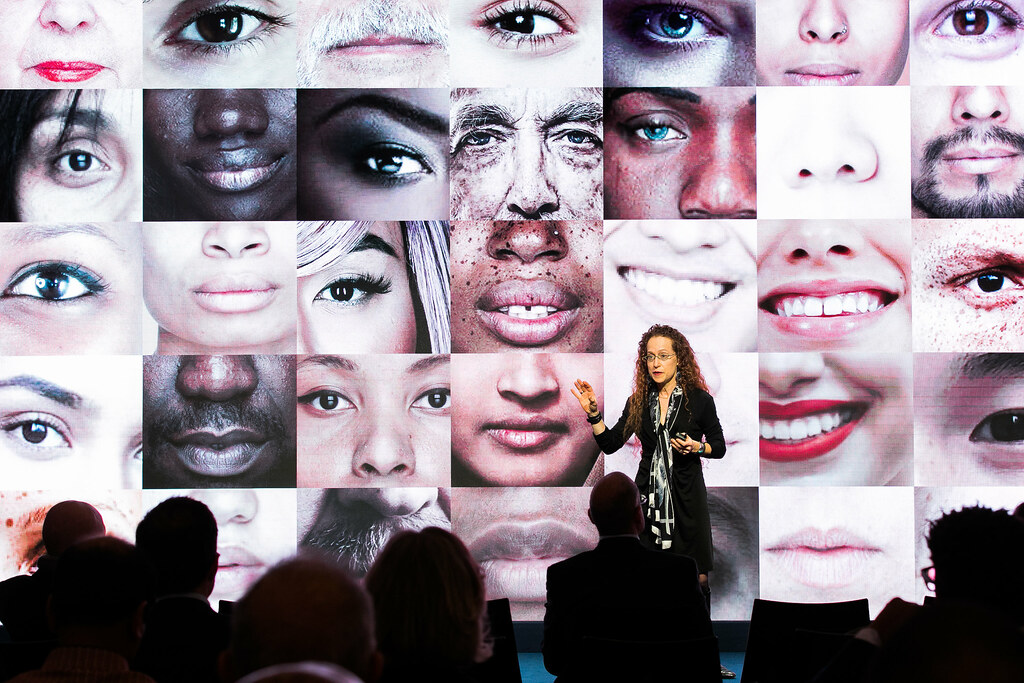
The program can be divided into two major areas:
- For the Government.
As mentioned above, a large part of the discrimination in the algorithm is due to the lack of diversity in policy formulation (Stevens & Keyes, 2021). Therefore, consideration should be given to policy formulation, such as inviting representatives from different regions and different ethnic groups to formulate policies on the basis of equal numbers of men and women.
- For the Platforms.
Platform supervision could improve these structural inequalities to a great extent as well. Appropriately excluding some pornographic, violent, threatening or terrorist content can purify the network environment to a certain extent and reduce structural inequality (Gillespie, 2018).
It still has a long way to go…
Obviously, the Internet has never been a utopia, and the structural inequalities that exists within the Internet has penetrated into all aspects of public life. Although the number of digital visits has increased significantly in recent years and the information received by the public has become more and more diverse, Internet still has major challenges to overcome. There is still a long way to go to reduce these inequalities, and there is a need for more policies or regulatory support.
References List
ADCU (2021). ADCU initiates legal action against Uber’s workplace use of racially discriminatory facial recognition systems. Retrieved from https://www.adcu.org.uk/news-posts/adcu-initiates-legal-action-against-ubers- workplace-use-of-racially-discriminatory-facial-recognition-systems
AI4People-An Ethical Framework for a Good AI Society. AI4People. Retrieved from https://www.eismd.eu/wp-content/uploads/2019/11/AI4People%E2%80%99s-Ethical-Framework-for-a-Good-AI-Society_compressed.pdf
Barrie, C. (2020). Searching Racism after George Floyd. Socius : Sociological Research for a Dynamic World, 6. https://doi.org/10.1177/237802312097150
FloridI, L., Cowls, J., Beltrametti, M., Chatila, R., Chazerand, P., Dignum, V., Luetge, C., Madelin, R., Pagallo, U., Rossi, F., Schafer, B., Valcke, P., & Vayena, E. (2019).
Gillespie, T. (2018). Custodians of the Internet. Yale University Press.
Hanrahan, C. (2020, December 2). Job recruitment algorithms can amplify unconscious bias favouring men, new research finds. ABC News. Retrieved from https://www.abc.net.au/news/2020-12-02/job-recruitment-algorithms-can-have-bias-against-women/12938870
Joohee, K., & Chang, J. (2021). Nth Room Incident in the Age of Popular Feminism: A Big Data Analysis. Azalea: Journal of Korean Literature & Culture, 14(14), 261-287.
Lusoli, A., & Turner, F. (2021). “It’s an Ongoing Bromance”: Counterculture and Cyberculture in Silicon Valley—An Interview with Fred Turner. Journal of Management Inquiry, 30(2), 235-242.
Magid, L. (2020, June 13). IBM, Microsoft and Amazon Not Letting Police Use Their Facial Recognition Technology. Forbes. Retrieved from https://www.forbes.com/sites/larrymagid/2020/06/12/ibm-microsoft-and-amazon-not-letting-police-use-their-facial-recognition-technology/?sh=3ff357c31887
Mariscal, J., Mayne, G., Aneja, U., & Sorgner, A. (2019). Bridging the gender digital gap. Economics, 13(1).
Martin, L. (2010). Africa and the Slave Trade (Black History). The School Librarian, 58(3), 185.
Noble, S. U. (2018). Algorithms of oppression. New York University Press.
Prates, M. O., Avelar, P. H., & Lamb, L. (2018). Assessing gender bias in machine translation–a case study with Google translate. arXiv preprint arXiv:1809.02208.
Stevens, N., & Keyes, O. (2021). Seeing infrastructure: race, facial recognition and the politics of data. Cultural Studies, 1-21.
United Nations Economic and Social Commission for Western Asia. (2020). Structural inequalities. https://archive.unescwa.org/structural-inequalities
Université de Montréal. (2018, December 5). The Montreal Declaration for the Responsible Development of Artificial Intelligence Launched. CABC. Retrieved from https://www.canasean.com/the-montreal-declaration-for-the-responsible-development-of-artificial-intelligence-launched/
Van Dijk, J., Poell, T., & De Waal, M. (2018). The platform society as a contested concept. The Platform Society. Public Values in a Connective World, 7-30.
The interaction between internet and structural inequalities by Estelle (Han) WANG is licensed under a Creative Commons Attribution-NonCommercial-ShareAlike 4.0 International License.